Sales Propensity Models: Not a Game of Chance
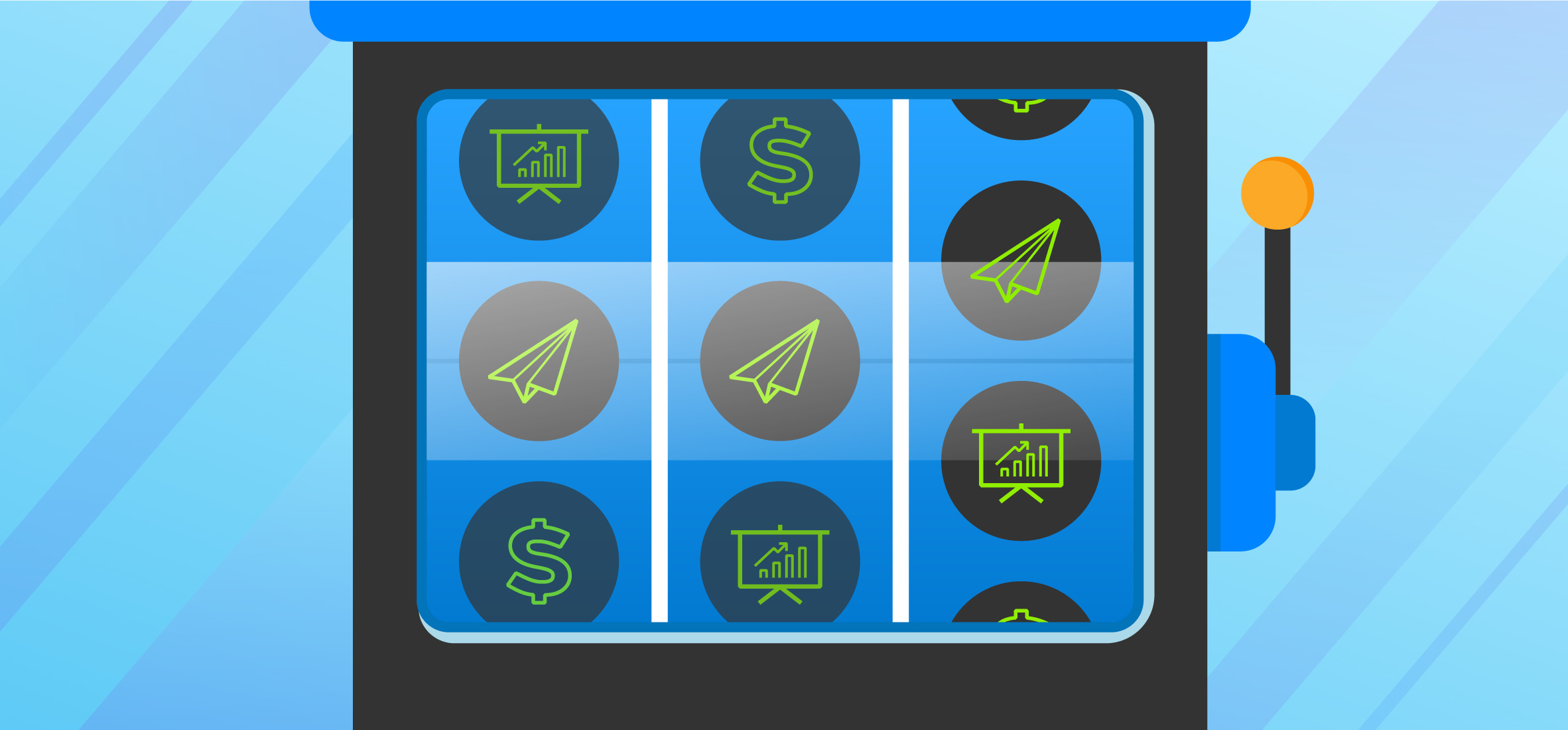
Most people, consciously or not, are constantly weighing their options. Is it better to stay on the freeway or take a side road during a traffic jam? What’s the probability that an item on your wish list will drop in price?
Sales programs are often the same way, relying on guesswork and gut instinct to determine such factors as the best time to contact customers, and the types of messaging and content that will hopefully pique their interest and move them through the sales funnel. Investing in data-driven marketing campaigns can certainly attract potential customers, but accurate knowledge about their purchase needs and timelines can be frustratingly elusive.
A common challenge in sales organizations is the sales prioritization problem that occurs when a business has many sales opportunities but a low overall conversion rate and finds pursuing prospects costly in terms of time and resources. Based on this scenario, the best course of action is to prioritize sales efforts to maximize revenues.
But the question is, how do you decide which opportunities to target and which ones to leave on the table?
Use what you know to predict what you don’t
It’s often said that human behavior is unpredictable, but research in psychology, neuroscience, and economics has consistently demonstrated just the opposite. One approach to prediction is to examine responses to stimuli, factors or events that evoke reactions. In marketing speak, stimuli are customer touchpoints.
Advances in data science have yielded powerful tools for the capture and analysis of these touchpoints, which might include filling out a lead card at a trade show, clicking on an online ad, downloading a brochure, or participating in a loyalty program.
Propensity models are a class of tools that support the prediction of sales outcomes from customer characteristics and their responses to touchpoints. The model identifies which customers are most likely to respond to specific sales activities, enabling sales organizations to focus their limited resources on the most promising opportunities.
This approach solves the sales prioritization problem by generating a predicted propensity-to-buy score for each opportunity. Sales prioritization simply becomes a matter of going down the list from highest to lowest propensity.
A strong propensity model is fed by high-quality data related to opportunities. Fortunately, most companies have historical data or touchpoints that can be used for predictive analytics to score leads as a part of their everyday business operations. This data can include firmographics (such as business size and number of employees), responses to marketing campaigns and events, and frequency and type of engagement.
What’s the payoff?
Many data-science tools are applicable for addressing questions about return on investment (ROI). To help clients understand the expected benefit of a propensity model, we’ve developed an ROI calculator that approximates the projected revenue from propensity-based prioritization. The estimator uses factors unique to a client’s business situation, such as average opportunity value (i.e., revenue per sale), estimated closure rate, and cost to explore each opportunity.
The ROI estimator compares the revenue produced by using a propensity model, which prioritizes sales, to that produced using random or no prioritization. If the client is currently using a data-driven prioritization solution, we’re also able to evaluate and compare that strategy, although, in our experience, most ad hoc solutions tend to perform similarly to random prioritization.
Implementing a propensity model
The process of developing and implementing a customized propensity model is typically a 12-week engagement, beginning with a detailed assessment of the quality and type of available foundational data. This data is first validated to determine its potential for predicting the quality of leads.
Next, we apply a rigorous scientific approach to ensure that only appropriate indicators are used as predictors in the model, thereby strengthening its performance.
This process often reveals valuable insight into the factors that impact sales success, factors that can then be used by operational groups to improve their sales and marketing programs.
Typically, a business’s existing data can be used to build a propensity model. However, collecting additional types of data can strengthen the model. For instance, campaign-engagement statistics may substantially increase the model’s predictive accuracy, leading to large gains in overall return on investment.
As part of our assessment, we also evaluate additional high-value data points that can improve the subsequent model.
During the model production and validation phase, we work closely with business subject-matter experts to ensure that the predictive structure makes sense in the context of the business and doesn’t rely on fickle indicators, as would be the case, for instance, in predicting outcome based on unusually successful sales teams or account owners.
Implementation of the model can take a variety of forms. Ideally, a propensity model is integrated with the client’s existing customer relationship management (CRM) platform as part of a holistic sales and marketing solution, one that provides valuable guidance and insight into customer relationships.
Once a model has been implemented, it is regularly refined and updated as further data is acquired, market conditions evolve, and the model converges with sales execution. We then continue to collaborate with our clients to validate the performance of their models.
Considering a propensity model? To see how this approach could benefit your sales organization, please check out our ROI calculator.
About The Author
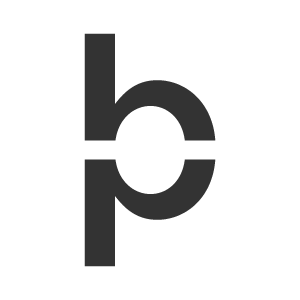